Sacha Epskamp Eiko I. In the case of regularized partial correlation networks the story is different.
Using a single line of code in our new R-package bootnet to estimate edge-weights accuracy you can now estimate the accuracy of the edge weights in the network leading to the.
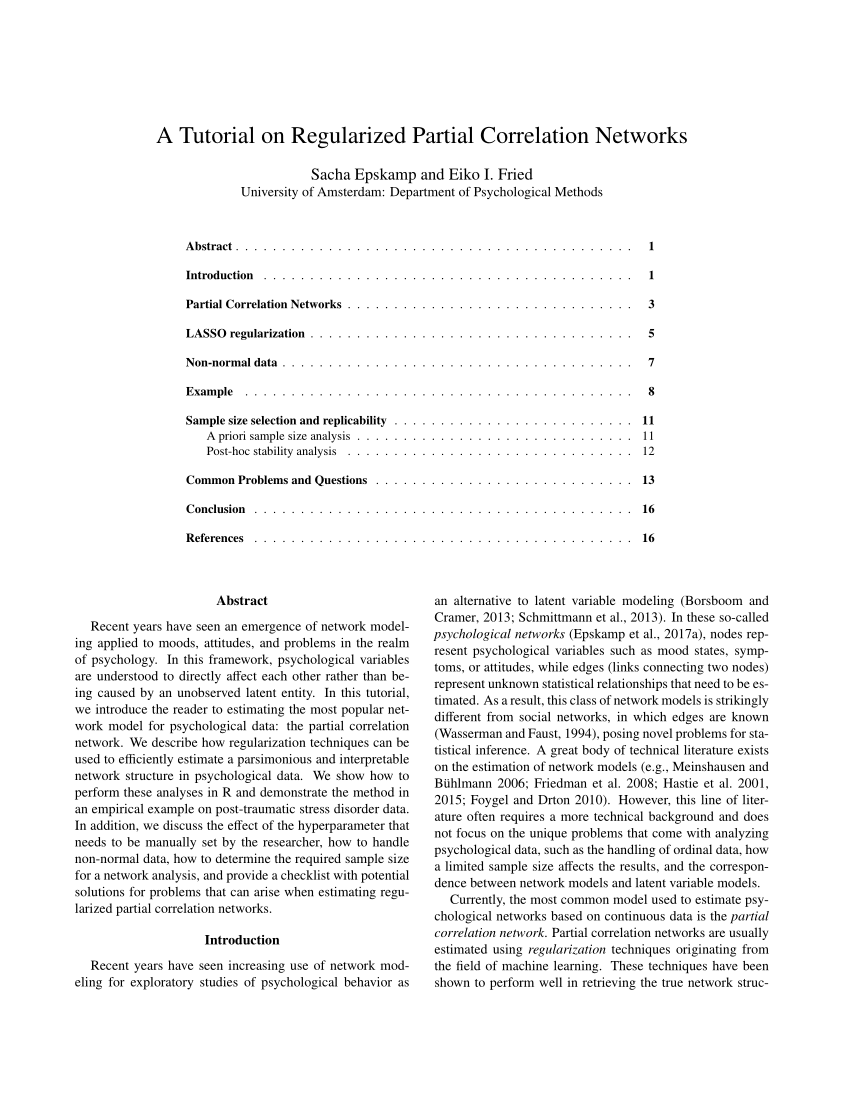
. Fried University of Amsterdam Abstract Recent years have seen an emergence of network modeling applied to moods attitudes and problems in the realm of psychology. Psychological Methods 234 617 - 634. Glasso networks regularized partial correlation networks were computed using the R qgraph package Epskamp Maris Waldorp Borsboom 2016.
In addition check out my published tutorials. The GGM can be estimated using regularization in combination with model selection using the extended Bayesian Information Criterion Foygel and Drton 2010. In this tutorial we introduce the reader to estimating the most popular network model for psychological data.
In this chapter we present a tutorial on estimating such regularized partial correlation networks using a methodology implemented. In this framework psychological variables are understood to directly affect each other. The third package SemNeT provides methods and measures for estimating and statistically comparing semantic networks via a point-and-click graphical user interface.
A Tutorial on Regularized Partial Correlation Networks. For this purpose we take a freely available dataset N359 and estimate a regularized partial correlation network in 17 PTSD symptoms which looks like this. In this tutorial we introduce the reader to estimating the most popular network model for psychological data.
The two correlation matrices are nearly perfectly linearly related with a correlation of 099. TitleA Tutorial on Regularized Partial Correlation Networks. We describe how regularization techniques can be used to efficiently estimate a parsimonious and interpretable network structure in psychological data.
A Tutorial on Regularized Partial Correlation Networks. We describe how regularization techniques can be used to efficiently estimate a parsimonious and interpretable network structure in psychological data. APA assumes no liability for errors or omissions and makes no.
AbstractRecent years have seen an emergence of network modeling applied to moodsattitudes and problems in the realm of psychology. In this frameworkpsychological variables are understood to directly interact with each otherrather than being caused by an unobserved latent entity. A Tutorial on Regularized Partial Correlation Networks Sacha Epskamp and Eiko I.
We show how to perform these analyses in R and demonstrate the method. This tutorial introduces the reader to estimating the most popular network model for psychological data. The partial correlation network.
In this tutorial we introduce the reader to estimating the most popular network model for psychological data. The partial correlation network. In recent literature the Gaussian Graphical model GGM.
This is a normal situation in statistics and the reason why we usually look at the CI coverage. Partial correlation networks are usually estimated using regularization an important statistical procedure that helps to recover the true network structure of the data. A Tutorial on Regularized Partial Correlation Networks.
This content was submitted by the author as supplemental material for an article published in APAs PsycARTICLES. If the CI includes 0 the parameter cannot be differentiated from 0. The partial correlation network.
In this tutorial we introduce the reader to estimating the most popular net- work model for psychological data. A Tutorial on Regularized Partial Correlation Networks. The content is presented as the author submitted it.
The partial correlation network. Fried Eiko I. Recent years have seen an emergence of network modeling applied to moods attitudes and problems in the realm of psychology.
Recent years have seen an emergence of network modeling applied to moods attitudes and problems in the realm of psychology. Epskamp S Borsboom D. The partial correlation network and describes how regularization techniques can be used to efficiently estimate a parsimonious and interpretable network structure in psychological data.
The indices of centrality also calculated with qgraph were used to assess the nature of the connections between nodes elements in the network. Psychological Methods 244 617 - 634. 20 rows This tutorial builds on the work of two prior tutorials.
In this tutorial weintroduce the reader to. In this framework psychological variables are understood to directly affect each other rather than being caused by an unobserved latent entity. Comparison of regularized partial correlation networks.
In this tutorial we introduce the reader to estimating the most popular network. In a second step we estimate regularized partial correlation networks Gaussian Graphical Models GGMs on the data. Estimating Psychological Networks and their Accuracy.
Lauritzen 1996 a network of partial correlation coefficients has been used to capture potential dynamic relationships between psychological variables. A Tutorial on Regularized Partial Correlation Networks. Regularized partial correlation network analyses with polychoric correlations suitable for ordinal data 19 were conducted to assess clustering of symptoms for the total sample including and excluding covariates and for each of the cancer types treatment regimens ie chemotherapy and radiotherapy yes or no and short-term and long-term.
Psychologische Methodenleer Psychologie FMG Date issued. If an edge is 01 after regularization that means we have two types of information about the parameter. Using real-world data we present a start-to-finish pipeline.
Pdf A Tutorial On Regularized Partial Correlation Networks Semantic Scholar
Pdf A Tutorial On Regularized Partial Correlation Networks
A Tutorial On Regularized Partial Correlation Networks Arxiv Vanity
A Tutorial On Regularized Partial Correlation Networks Arxiv Vanity
0 comments
Post a Comment